
This event has passed.
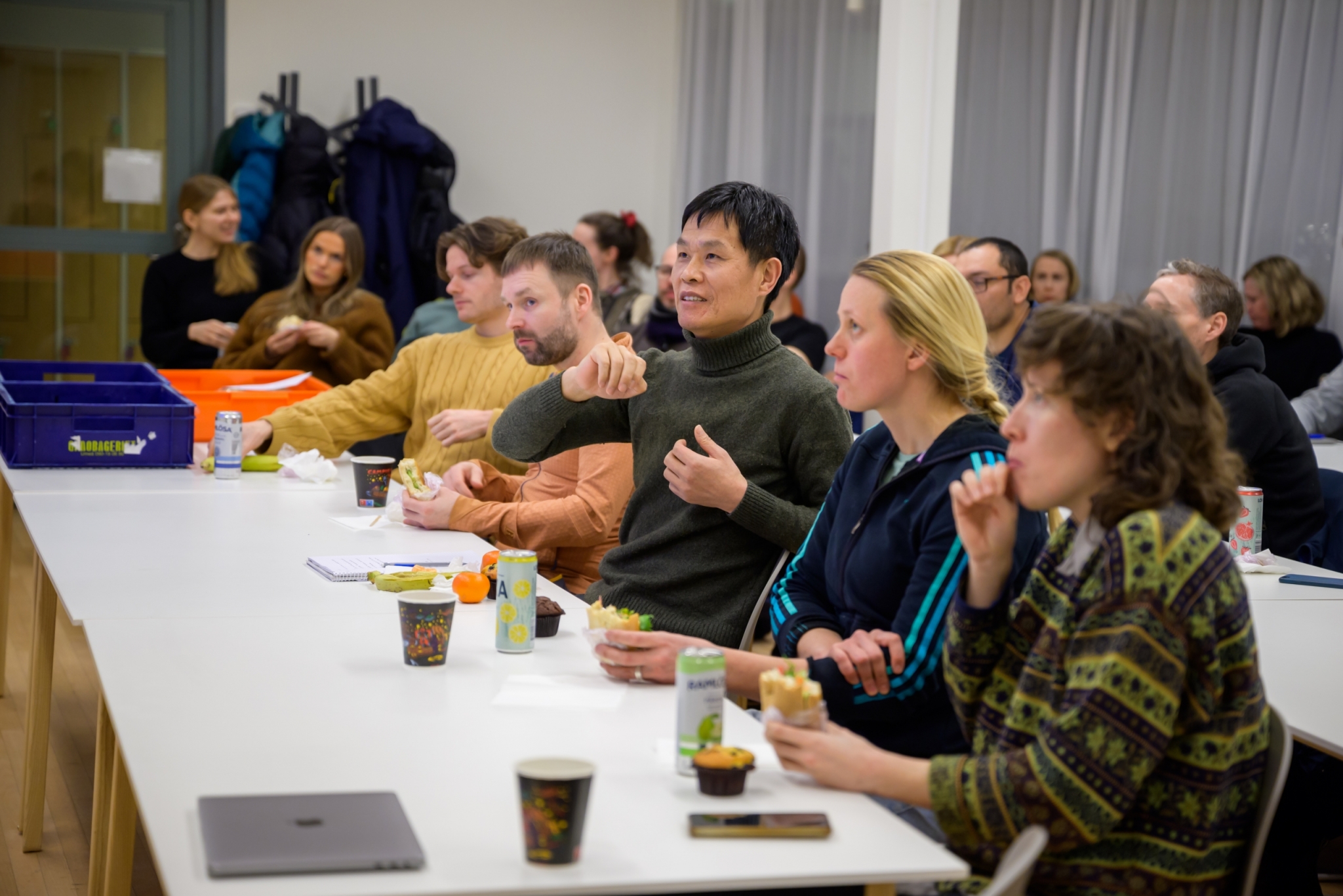
Lunch Pitch with Paolo Bientinesi; Beatrice Melin, Minh Hoang Vu, Wilfred de Graaf
May 8 @ 12:00 - 13:00
Lunch Pitches with Paolo Bientinesi and Beatrice Melin with Minh Hoang Vu and Wilfred de Graaf
To encourage cross pollination of ideas between researchers from different disciplines, IceLab hosts interdisciplinary research lunches with the vision of allowing ideas to meet and mate. During the Lunch Pitch Season, the creative lunches take place at KBC on a Wednesday.
Place: KBC Glasburen
Time: Wednesday 8 May at 12:00.
Pitch 1: Paolo Bientinesi: Importance and limitations of software libraries in computational
workflows
Professor at Department of Computing ScienceAbstract:
Traditionally, software libraries are a collection of functions, each designed to support one specific mathematical operation. A rigid interface allows users to invoke a function, and provide both input arguments and algorithmic parameters. The benefits of such a design are numerous, e.g., separation of concerns, possibility of optimization, portability, and more. However, there are situations in which such libraries are undesirable. In many scientific applications, not one, but many problems of the same type need to be solved. The specific scenario that I will discuss is when those problems are correlated in some way with one another, i.e., they form a “sequence”. Examples include linear systems in which the coefficient matrix evolves parametrically, or problems that share partially the input data. Depending on the nature of the correlation, the solution of the entire sequence can be much faster than the solution of the individual problems.
Pitch 2 & 3: Beatrice Melin with Minh Hoang Vu and Wilfred de Graaf: From catching criminals to catching cancer – can anomali models be used in health data?
Beatrice Melin, Professor, senior consultant (attending) physician at Department of Diagnostics and InterventionMinh Hoang Vu, Postdoctoral fellow at IceLab and Department of Diagnostics and Intervention
Wilfred de Graaf, Heights.ai partner
Abstract:
Glioma, malignant brain tumors are a challenging tumor as few treatment options currently exist. The diagnosis is complex, there are several subgroups and no common life style factors have been linked to the causes of brain tumors. Diagnostics is often performed after severe symptoms by MRI scans and neurosurgery, and tumors are therefore often detected at a late stage. Our research groups tries to understand how glioma develops to be able to find methods for prevention, early detection and novel target for treatment. One question is therefore if there are novel approaches to data analyses that could help us find brain tumors early, using health data from medical records and maybe some blood biomarkers? As glioma is rare, we want to collaborate globally to be able to have large enough studies and also robust risk factors that applies to many populations. The difficulty is finding ways to share data considering data privacy. One way of sharing data is to generate synthetic data that could be used for analyses. The second question is therefore if synthetic data could be of high quality enough to enable global collaboration? Are there some AI models that can be used on synthetic data and others need real world data? We are hoping for a fruitful discussion to gain insights and novel methods in our projects.
Interested in: Discussing models that work robustly to detect rare disease in health data. All input is welcomed. The research group has vast experience of analysing health data in tradional ways. What benefits can AI models do?